DATE
13th April 2025
CATEGORY
Accounting and Finance, Artificial Intelligence, Business Strategy
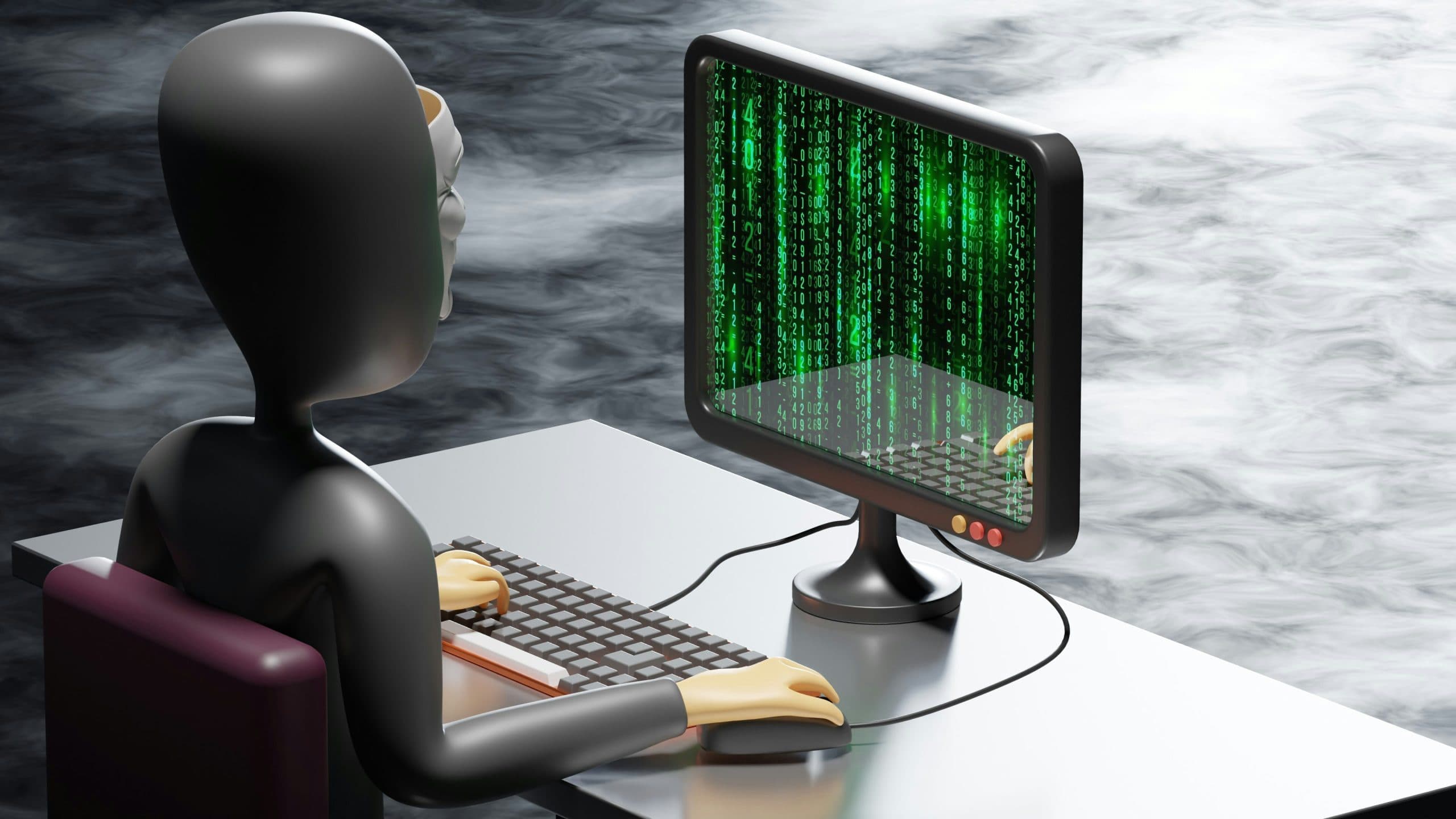
Understanding AI in Fraud Detection
AI, or Artificial Intelligence, is revolutionizing numerous sectors, and the financial industry is no exception. In essence, AI encompasses machine learning, natural language processing, and other technologies that empower systems to mimic human behavior and decision-making. For financial institutions, leveraging AI for fraud detection is vital as it can analyze patterns in data at a scale and speed unattainable by humans.
Traditionally, fraud detection relied on rule-based systems where predefined criteria determined “suspicious” activities. These methods involve significant manual efforts and can often yield inaccurate results, leaving gaps for fraudsters to exploit.
Nonetheless, conventional approaches are limited in their scope. They tend to overlook evolving fraudulent techniques and are often plagued by high false-positive rates, leading to unnecessary investigations and operational inefficiencies.
AI enhances detection capabilities by analyzing vast datasets in real-time, identifying unusual patterns and anomalies that might indicate fraudulent activity. By learning from data continuously, AI systems can adapt and refine their algorithms, significantly improving detection accuracy.
Pro Tip: To get started with AI in fraud detection, invest in high-quality data collection and cleaning processes to ensure your AI has the best possible foundation to learn from.
The Benefits of AI-Based Fraud Detection Systems
Implementing AI not only enhances fraud detection but also offers several compelling advantages for financial institutions. The accuracy of identifying fraudulent transactions skyrockets with machine learning algorithms that analyze behaviors and flag anomalies.
Moreover, AI significantly reduces false positives, which historically burden fraud investigation teams. By minimizing unnecessary alerts, institutions can streamline workflows and focus efforts on genuine threats.
Real-time monitoring is another critical benefit. AI-enabled systems can detect and respond to fraudulent activities as they happen, allowing financial institutions to mitigate risks immediately.
Lastly, the cost savings achieved through AI-based systems can be substantial. Automated processes reduce manpower needed for investigations, translating to significant reductions in fraud management costs.
Pro Tip: Regularly evaluate the performance metrics of your AI systems to capitalize on ongoing improvements, ensuring they evolve alongside new fraud tactics.
Key Components the Implementation
To successfully implement AI in fraud detection, certain components must be in place. First, data quality is paramount. Integrating data from various sources—including transactional, behavioral, and demographic—is crucial for effective analysis.
Secondly, leveraging robust machine learning algorithms is essential. Organizations should prioritize consistent model training to refine their predictive capabilities continually.
Additionally, developing an adaptive AI system is non-negotiable. The landscape of fraud is always changing, and your AI must evolve to keep pace with new tactics.
Finally, maintaining transparent AI models is vital for compliance. Financial institutions need to demonstrate the logic behind decisions made by AI systems to adhere to regulatory standards.
Pro Tip: Engage with data scientists and AI experts during the integration process to ensure a holistic approach and cultivate a culture of data-driven decision-making.
Steps for Implementing AI-Based Fraud Detection Systems
The path to implementing AI-based fraud detection systems begins with evaluating existing fraud processes. Identify the gaps where traditional methods fall short, allowing you to tailor AI solutions effectively.
Next, selecting the right technology and partners is crucial. A strong collaboration with technology providers that specialize in AI can aid in navigating complexities in the development phase.
Planning a phased implementation strategy is advisable. Start small, measure outcomes, and expand your solutions gradually, thus reducing disruption in operations.
Finally, training staff to understand and interpret AI-driven insights will empower them. This ensures that your organization can leverage the full potential of the technology.
Pro Tip: Establish a feedback loop post-implementation; this helps in continually refining AI solutions based on user experience and changing fraud patterns.
Challenges in Implementing AI in Fraud Detection
While implementing AI offers vast benefits, challenges remain. Data privacy concerns are paramount, as compliance with regulations like GDPR and CCPA is critical to avoid hefty fines.
Resistance to change is another common hurdle. Employees accustomed to traditional methods may struggle with the transition to AI, necessitating comprehensive change management strategies.
Furthermore, biases in AI algorithms can lead to adverse outcomes, potentially resulting in unfair treatment of certain groups. Thus, continuous monitoring and adjustments are essential.
Lastly, ensuring system security against potential exploits by fraudsters remains a challenge. Vigilant security measures must be in place to protect the integrity of the AI models.
Pro Tip: Foster a culture of awareness and adaptability in your workforce to pave the way for smoother transitions and more efficient AI application.
Real-World Case Studies and Success Stories
Many leading financial institutions have already harnessed AI for fraud detection with remarkable success. For example, Bank of America implemented machine learning, resulting in a 60% decrease in false positives in their fraud detection model.
Metrics post-implementation often showcase reduced fraud rates, with institutions reporting significant savings and improved operational efficiency. However, some organizations have faced challenges, serving as cautionary tales for others.
By learning from these experiences, companies can embrace best practices, such as focusing on cross-departmental collaboration and investing in continuous training programs.
Pro Tip: Regularly share success stories and lessons learned within your organization to inspire confidence and enthusiasm for new technologies.
Future Trends in AI and Fraud Detection
The future promises exciting developments in AI and fraud detection. Advanced analytics and big data will elevate AI systems, enhancing their efficacy in detection and prediction.
Integrating AI with blockchain technologies stands as a promising avenue. The transparency and security inherent in blockchain can reinforce AI detection systems, adding another layer of security.
Moreover, the rise of collaborative fraud detection networks among financial institutions can prove beneficial. Sharing data and insights collectively may lead to a more comprehensive understanding of fraudulent activities.
Lastly, predictive analytics will usher in the next generation of fraud prevention strategies, utilizing historical data to foresee and mitigate potential threats.
Pro Tip: Stay informed about emerging technologies and trends in AI to position your institution ahead of industry changes and challenges.